Unlocking the Power of Image Datasets for Object Detection
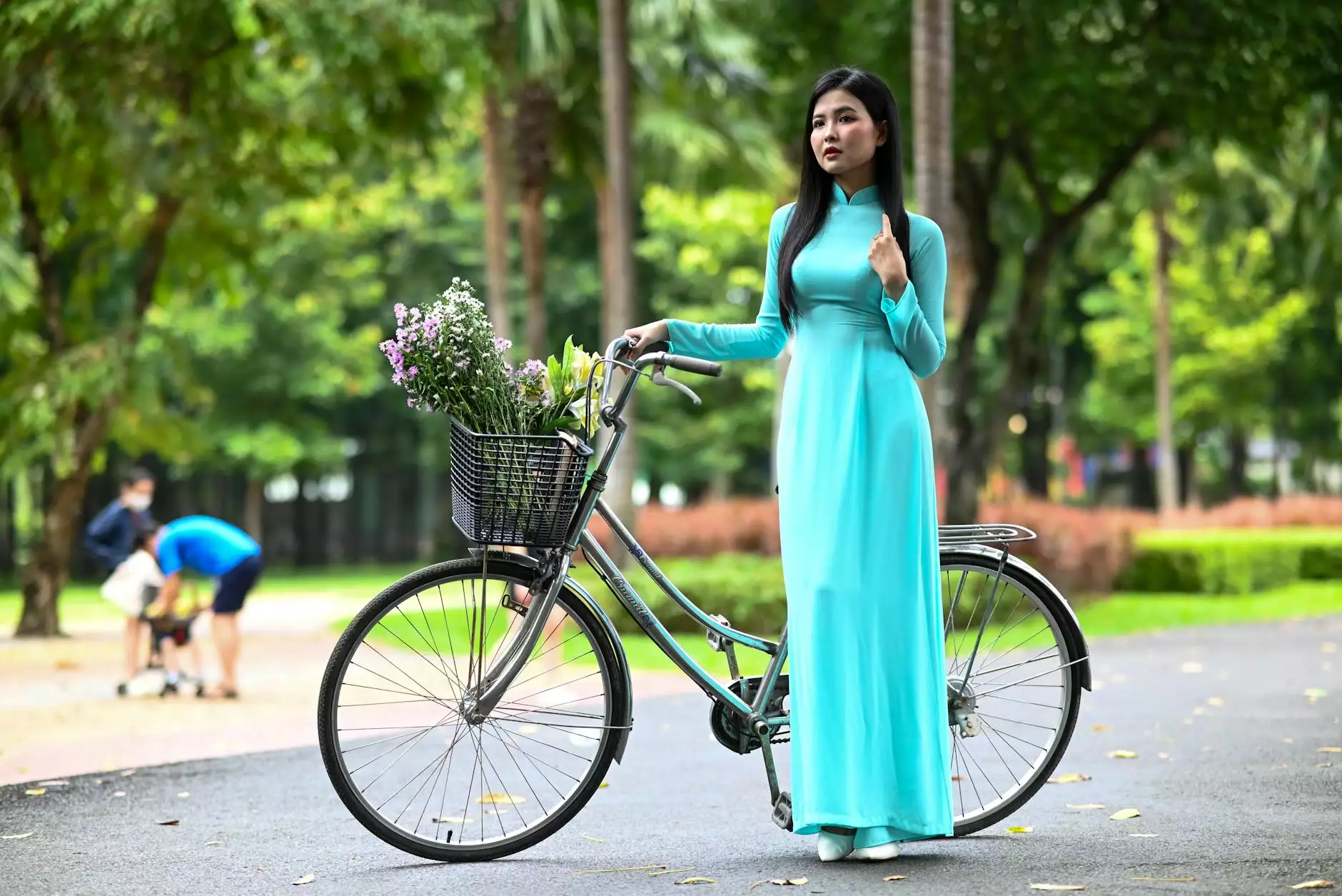
In the realm of artificial intelligence and machine learning, image datasets for object detection have emerged as a cornerstone in training models to accurately identify and classify various objects in visual data. As industries increasingly lean on AI-driven solutions, understanding the significance and methodology behind these datasets has never been more vital. This article delves deep into how robust datasets, combined with advanced data annotation tools and platforms, unlock new levels of accuracy and efficiency in object detection systems.
The Importance of Image Datasets
Image datasets serve as the backbone of training and validating object detection algorithms. The quality and quantity of images used directly affect the model's performance and reliability. Here’s why they matter:
- Variability: A diverse dataset with various objects, backgrounds, and contexts enables models to generalize better across real-world scenarios.
- Annotation Quality: Precise annotations that correctly indicate object locations and categories are crucial for training effective models.
- Volume: Large datasets help in reducing overfitting—where a model performs well on training data but poorly on unseen data.
Data Annotation Tools: Elevating Image Datasets for Object Detection
To leverage the full potential of image datasets, data annotation tools play an integral role. KeyLabs.ai offers state-of-the-art solutions tailored for high-fidelity object detection tasks.
Key Features of Data Annotation Tools
Here are several key features that make effective data annotation tools indispensable in the process:
- Automation: The incorporation of AI-driven automation simplifies repetitive tasks and speeds up the annotation process.
- Collaboration: Facilitate teamwork by allowing multiple users to annotate simultaneously and review each other's work effortlessly.
- Quality Control: Built-in quality assurance mechanisms ensure that the annotations are accurate and consistent.
- Integration: Seamless integration capabilities with machine learning frameworks and data management tools enhance workflow efficiency.
Building an Effective Image Dataset for Object Detection
The creation of a high-quality image dataset is a meticulous process that involves several steps:
Step 1: Image Collection
The first step in creating an effective image dataset is image collection. This process involves:
- Gathering images from diverse sources, including public datasets, social media, and proprietary collections.
- Ensuring images represent a wide variety of scenarios, lighting conditions, and object placements.
- Maintaining copyright compliance and ensuring permission for usage.
Step 2: Annotation
Once images are collected, the next step is annotation. This is where the data annotation tools come into play:
Utilizing advanced tools like those offered by KeyLabs.ai, teams can annotate images by:
- Drawing bounding boxes around objects to identify their locations.
- Labeling each box with the correct object category, ensuring models learn to recognize various items accurately.
- Using segmentation techniques for more precise object boundaries in complex images.
Step 3: Validation
The final step involves validating the dataset. This crucial phase guarantees the quality and reliability of the dataset:
- Conducting reviews of annotated images for accuracy and consistency.
- Implementing a feedback system to continuously improve the annotation process.
Object Detection: Techniques and Algorithms
With effective image datasets at the core, various techniques and algorithms can be applied to achieve successful object detection.
Popular Algorithms
Some of the most popular algorithms in object detection include:
- YOLO (You Only Look Once): A single-stage detection algorithm that processes images extremely quickly.
- Faster R-CNN: Combines region proposal networks with convolutional networks for higher accuracy.
- SSD (Single Shot MultiBox Detector): Balances speed and accuracy by detecting objects in a single pass.
Real-World Applications of Object Detection
Object detection powered by robust image datasets has found applications across numerous industries:
1. Healthcare
In healthcare, object detection can assist in identifying tumors in medical imaging and tracking patient health through wearable devices.
2. Automotive
Autonomous vehicles rely on object detection to recognize pedestrians, traffic signs, and other vehicles on the road.
3. Retail
Retailers use object detection for inventory tracking, customer behavior analysis, and enhancing shopping experiences.
4. Agriculture
In agriculture, crop monitoring and pest detection through drone imagery enable more efficient farming practices.
Enhancing Object Detection with Quality Datasets
Enhancing the performance of your object detection applications relies heavily on the quality of the image datasets you utilize. Here are several strategies to improve your datasets:
1. Data Augmentation
Data augmentation techniques, such as rotating images, scaling, and changing brightness, help in creating synthetically diverse datasets. This not only enhances the learning of models but also counteracts overfitting.
2. Continuous Data Updates
Regularly updating your dataset with new images ensures the model remains relevant and accurate in changing environments.
3. User Feedback Integration
Gathering feedback from users who interact with the object detection system can provide insights into areas needing improvement, allowing for dataset refinement.
The Future of Object Detection and Data Annotation
The future of object detection lies in continuous advancement in machine learning algorithms and data annotation techniques. As AI technology evolves, we will likely see:
- Improved Automation: Further enhancement in AI tools to automate data labeling, making processes faster and cheaper.
- More Sophisticated Models: Models capable of understanding more complex relationships between objects and scenes.
- Greater Accessibility: Tools and platforms like KeyLabs.ai making high-quality data annotation accessible to more businesses.
Conclusion
In conclusion, the effectiveness of image datasets for object detection cannot be overstated. Leveraging sophisticated data annotation tools and platforms like KeyLabs.ai not only enhances data quality but also empowers industries to adopt AI solutions effectively. As the demand for accurate and reliable object detection systems grows, so too will the necessity for high-quality datasets. Investing in these resources is not just an option—it’s a necessity for future success.
© 2023 KeyLabs.ai - Transforming Data Annotation for a Smarter Future