Maximizing Efficiency with Image Annotation Tools for Machine Learning
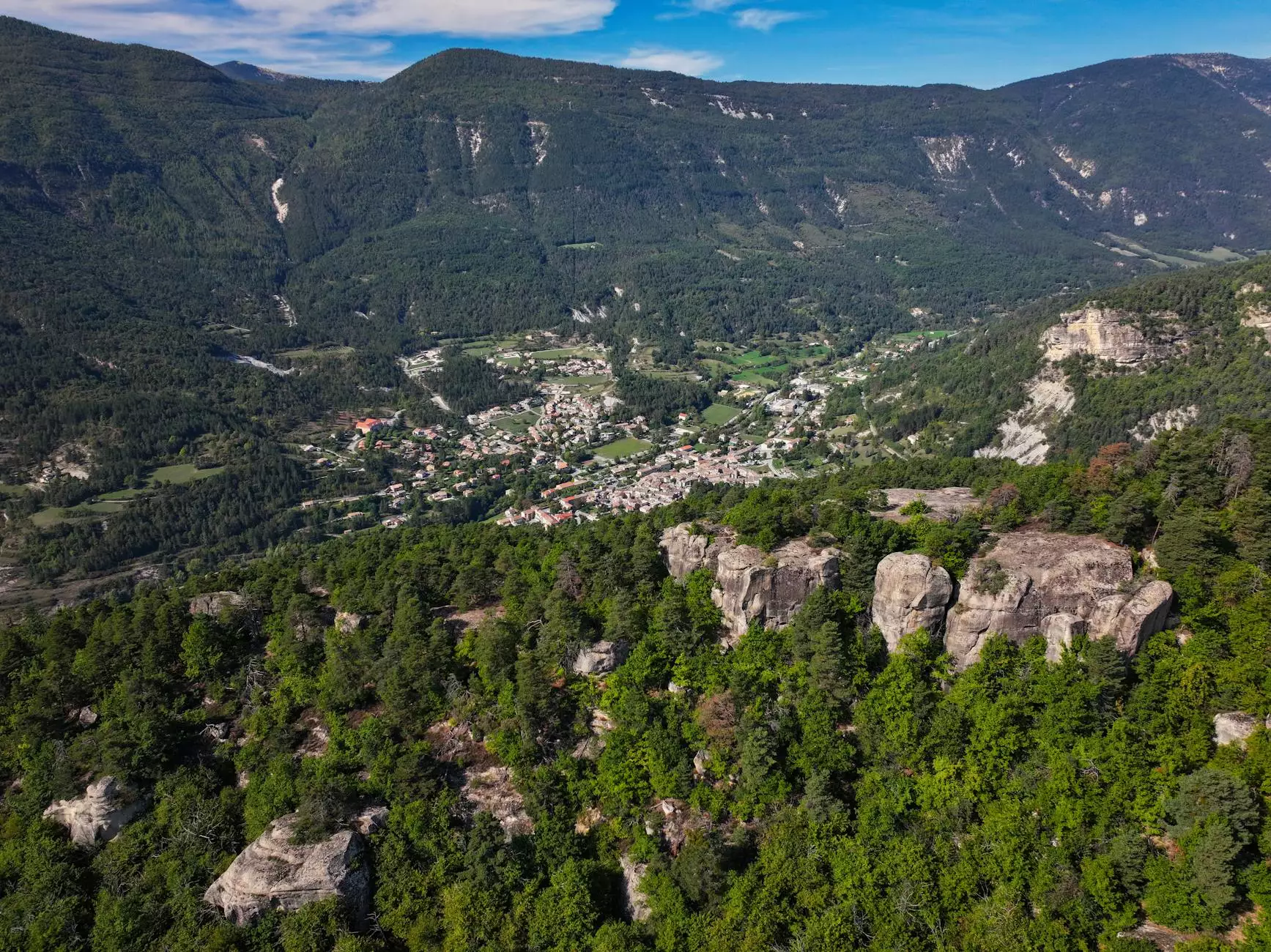
In today's fast-paced technological landscape, businesses must continuously innovate to stay ahead. One of the key drivers of innovation is data, and in machine learning, data quality is paramount. This is where image annotation tools for machine learning come into play. These tools not only enhance the quality of data used in training models but also streamline the entire processes involved in annotating and labeling images. In this article, we will explore the significance of data annotation, how these tools function, and their immense benefits in various industries.
Understanding Data Annotation
Data annotation is the process of labeling data to make it understandable for machine learning algorithms. In the realm of image processing, this means tagging various elements within images so that machine learning models can learn from them. The main goal is to provide machines with the ability to recognize objects, classify images, and even connect visual cues with actions and responses.
There are several forms of image annotation, including:
- Bounding Boxes: Drawing boxes around objects in an image is one of the most common forms of annotation.
- Semantic Segmentation: This involves labeling every pixel of an image, which helps in understanding the context more deeply.
- Keypoint Annotation: It involves annotating specific points within an image to indicate features, useful in fields like pose estimation.
- Polygon Annotation: For more complex shapes, polygons are used to annotate objects accurately.
The Evolution of Image Annotation Tools
With the evolution of machine learning and artificial intelligence, the demand for sophisticated image annotation tools has surged. Earlier methods were labor-intensive, relying on manual processes that were often prone to errors. However, modern tools leverage automation, artificial intelligence and collaborative frameworks to enhance efficiency and accuracy.
These advanced tools often include features such as:
- User-Friendly Interfaces: To facilitate easy navigation and operation, a good annotation tool provides an intuitive interface.
- Integration Capabilities: Ability to integrate with existing data management systems and machine learning frameworks.
- Collaboration Features: Allow teams to work together seamlessly, enabling real-time feedback and improvements.
- Quality Control Mechanisms: Ensuring that the annotated data meets the required standards before being utilized.
Benefits of Using Image Annotation Tools for Machine Learning
The implementation of image annotation tools for machine learning can transform your business operations significantly. Let's delve into some of the key benefits:
1. Improved Accuracy of Machine Learning Models
High-quality annotated data ensures that machine learning models are trained effectively, leading to improved predictions and outcomes. Annotating data accurately minimizes biases and maximizes learning potential.
2. Enhanced Productivity
With automated annotation tools, the time required to process images is drastically reduced compared to manual methods. This allows businesses to focus their resources on developing and optimizing their AI models rather than getting bogged down by data preparation tasks.
3. Scalability
As businesses grow, so does their data. Efficient annotation tools allow for scalability without compromising quality. Whether you need to annotate thousands or millions of images, advanced tools can handle the increase in workload seamlessly.
4. Cost-Effective Solutions
Investing in an image annotation tool can lead to significant cost savings. By automating many processes, organizations can reduce labor costs and minimize errors that could lead to expensive rework.
5. Versatility Across Industries
From healthcare to automotive, retail to agriculture, image annotation is relevant across various sectors. Different industries can leverage these tools to extract insights from images, whether it's identifying medical conditions in radiology images or automating quality control in manufacturing.
Choosing the Right Data Annotation Platform
When selecting a data annotation platform, businesses should consider several critical factors to ensure that they choose the right tool for their specific needs:
1. Feature Set
Evaluate the features that the platform offers. Does it support the type of annotations you need? Are there options for quality control? Consider tools that offer flexibility for various annotation types.
2. User Experience
An intuitive user interface can significantly affect the productivity of your team. The easier it is for your team to navigate the tool, the faster and more efficiently they can annotate data.
3. Collaboration Capabilities
In a team environment, collaboration features should be a priority. Look for tools that allow team members to share feedback, communicate effectively, and work together on annotations seamlessly.
4. Integration with Existing Workflows
Your image annotation tool should integrate smoothly with your existing workflows and systems, whether they are data storage solutions, machine learning platforms, or project management tools.
5. Customer Support and Resources
Consider the level of support and resources available. Comprehensive documentation, training sessions, and responsive customer service can be invaluable, especially when onboarding new tools.
Best Practices for Using Image Annotation Tools
To maximize the effectiveness of image annotation tools for machine learning, it’s essential to follow best practices in the annotation process:
1. Define Clear Guidelines
Before beginning the annotation process, it’s vital to develop clear and detailed guidelines for annotators. This ensures consistency and quality across the annotated data.
2. Utilize Experienced Annotators
While automation is an asset, human oversight is crucial. Engaging experienced annotators can ensure that complex annotations are correctly executed and fine-tuned.
3. Implement Regular Quality Checks
Incorporate regular quality assurance checks to maintain high standards in data annotations. Randomly sampled reviews of the annotated data can help in identifying and correcting errors.
4. Keep Learning and Iterating
Machine learning is an ever-evolving field. Encourage your team to stay updated on the latest trends and technologies in data annotation to continually improve processes.
Case Studies and Real-World Applications
To contextualize the importance of image annotation tools for machine learning, let’s explore a few case studies that highlight their impact:
Health Sector: Radiology and Medical Imaging
In healthcare, annotated medical images are critical for training diagnostic algorithms. A leading healthcare provider utilized a data annotation platform to label thousands of radiology images. The result was a machine learning model that significantly improved the accuracy of identifying tumors, which ultimately enhanced patient outcomes.
Automotive: Self-Driving Technology
The automotive industry is one of the most affected by advancements in machine learning. Companies developing autonomous vehicles rely on precise image annotations for recognizing objects like pedestrians, road signs, and other vehicles. By employing a robust data annotation platform, these companies have managed to train their models more efficiently, leading to safer driving environments.
Agriculture: Crop Monitoring and Management
Farmers and agri-tech companies can benefit immensely from image annotations for crop monitoring using drones. By annotating images of farmland with varying crop health statuses, machine learning models can predict yields and suggest optimal farming practices. This not only boosts productivity but also enhances sustainability.
Conclusion: Embrace the Future with Image Annotation
The integration of image annotation tools for machine learning into business processes represents a transformative step towards leveraging data for competitive advantage. As industries continue to undergo digital transformations, the ability to annotate and utilize image data efficiently will set organizations apart in the race for innovation. With proper implementation and a focus on quality, businesses can harness the power of machine learning to unlock new possibilities and drive growth.
At KeyLabs.ai, we provide comprehensive data annotation tools and platforms designed to elevate your machine learning initiatives. Embrace innovation with our sophisticated solutions and watch your business transform.
image annotation tool machine learning